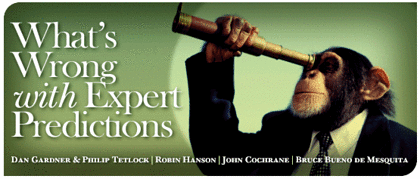
About this Issue
Experts must love making predictions. They keep right on predicting, even though by any reasonable standard, they’re terrible at it.
Many of them, though intelligent and well-informed, nonetheless have difficulty even beating a random guess about future events—or, if you will, beating the proverbial dart-throwing chimp. This applies to many realms of human activity, but above all to politics, and the subject of expert political judgment forms this month’s theme at Cato Unbound.
Once we grasp that the experts aren’t so reliable at predicting the future, a question arises immediately: How can we do better? Some events will always be unpredictable, of course, but this month’s lead authors, Dan Gardner and Philip E. Tetlock, suggest a few ways that the experts might still be able to improve.
To discuss with them, we’ve invited economist and futurologist Robin Hanson of George Mason University, Professor of Finance and Cato Adjunct Scholar John H. Cochrane, and political scientist Bruce Bueno de Mesquita. Each will offer a commentary on Gardner and Tetlock’s essay, followed by a discussion among the panelists lasting through the end of the month.
Lead Essay
Overcoming Our Aversion to Acknowledging Our Ignorance
Each December, The Economist forecasts the coming year in a special issue called The World in Whatever-The-Next-Year-Is. It’s avidly read around the world. But then, like most forecasts, it’s forgotten.
The editors may regret that short shelf-life some years, but surely not this one. Even now, only halfway through the year, The World in 2011 bears little resemblance to the world in 2011. Of the political turmoil in the Middle East—the revolutionary movements in Tunisia, Egypt, Libya, Yemen, Bahrain, and Syria—we find no hint in The Economist’s forecast. Nor do we find a word about the earthquake/tsunami and consequent disasters in Japan or the spillover effects on the viability of nuclear power around the world. Or the killing of Osama bin Laden and the spillover effects for al Qaeda and Pakistani and Afghan politics. So each of the top three global events of the first half of 2011 were as unforeseen by The Economist as the next great asteroid strike.
This is not to mock The Economist, which has an unusually deep bench of well-connected observers and analytical talent. A vast array of other individuals and organizations issued forecasts for 2011 and none, to the best of our knowledge, correctly predicted the top three global events of the first half of the year. None predicted two of the events. Or even one. No doubt, there are sporadic exceptions of which we’re unaware. So many pundits make so many predictions that a few are bound to be bull’s eyes. But it is a fact that almost all the best and brightest—in governments, universities, corporations, and intelligence agencies—were taken by surprise. Repeatedly.
That is all too typical. Despite massive investments of money, effort, and ingenuity, our ability to predict human affairs is impressive only in its mediocrity. With metronomic regularity, what is expected does not come to pass, while what isn’t, does.
In the most comprehensive analysis of expert prediction ever conducted, Philip Tetlock assembled a group of some 280 anonymous volunteers—economists, political scientists, intelligence analysts, journalists—whose work involved forecasting to some degree or other. These experts were then asked about a wide array of subjects. Will inflation rise, fall, or stay the same? Will the presidential election be won by a Republican or Democrat? Will there be open war on the Korean peninsula? Time frames varied. So did the relative turbulence of the moment when the questions were asked, as the experiment went on for years. In all, the experts made some 28,000 predictions. Time passed, the veracity of the predictions was determined, the data analyzed, and the average expert’s forecasts were revealed to be only slightly more accurate than random guessing—or, to put more harshly, only a bit better than the proverbial dart-throwing chimpanzee. And the average expert performed slightly worse than a still more mindless competition: simple extrapolation algorithms that automatically predicted more of the same.
Cynics resonate to these results and sometimes cite them to justify a stance of populist know-nothingism. But we would be wrong to stop there, because Tetlock also discovered that the experts could be divided roughly into two overlapping yet statistically distinguishable groups. One group would actually have been beaten rather soundly even by the chimp, not to mention the more formidable extrapolation algorithm. The other would have beaten the chimp and sometimes even the extrapolation algorithm, although not by a wide margin.
One could say that this latter cluster of experts had real predictive insight, however modest. What distinguished the two groups was not political ideology, qualifications, access to classified information, or any of the other factors one might think would make a difference. What mattered was the style of thinking.
One group of experts tended to use one analytical tool in many different domains; they preferred keeping their analysis simple and elegant by minimizing “distractions.” These experts zeroed in on only essential information, and they were unusually confident—they were far more likely to say something is “certain” or “impossible.” In explaining their forecasts, they often built up a lot of intellectual momentum in favor of their preferred conclusions. For instance, they were more likely to say “moreover” than “however.”
The other lot used a wide assortment of analytical tools, sought out information from diverse sources, were comfortable with complexity and uncertainty, and were much less sure of themselves—they tended to talk in terms of possibilities and probabilities and were often happy to say “maybe.” In explaining their forecasts, they frequently shifted intellectual gears, sprinkling their speech with transition markers such as “although,” “but,” and “however.”
Using terms drawn from a scrap of ancient Greek poetry, the philosopher Isaiah Berlin once noted how, in the world of knowledge, “the fox knows many things but the hedgehog knows one big thing.” Drawing on this ancient insight, Tetlock dubbed the two camps hedgehogs and foxes.
The experts with modest but real predictive insight were the foxes. The experts whose self-concepts of what they could deliver were out of alignment with reality were the hedgehogs.
It’s important to acknowledge that this experiment involved individuals making subjective judgements in isolation, which is hardly the ideal forecasting method. People can easily do better, as the Tetlock experiment demonstrated, by applying formal statistical models to the prediction tasks. These models out-performed all comers: chimpanzees, extrapolation algorithms, hedgehogs, and foxes
But as we have surely learned by now—please repeat the words “Long Term Capital Management”—even the most sophisticated algorithms have an unfortunate tendency to work well until they don’t, which goes some way to explaining economists’ nearly perfect failure to predict recessions, political scientists’ talent for being blindsided by revolutions, and fund managers’ prodigious ability to lose spectacular quantities of cash with startling speed. It also helps explain why so many forecasters end the working day with a stiff shot of humility.
Is this really the best we can do? The honest answer is that nobody really knows how much room there is for systematic improvement. And, given the magnitude of the stakes, the depth of our ignorance is surprising. Every year, corporations and governments spend staggering amounts of money on forecasting and one might think they would be keenly interested in determining the worth of their purchases and ensuring they are the very best available. But most aren’t. They spend little or nothing analyzing the accuracy of forecasts and not much more on research to develop and compare forecasting methods. Some even persist in using forecasts that are manifestly unreliable, an attitude encountered by the future Nobel laureate Kenneth Arrow when he was a young statistician during the Second World War. When Arrow discovered that month-long weather forecasts used by the army were worthless, he warned his superiors against using them. He was rebuffed. “The Commanding General is well aware the forecasts are no good,” he was told. “However, he needs them for planning purposes.”
This widespread lack of curiosity—lack of interest in thinking about how we think about possible futures—is a phenomenon worthy of investigation in its own right. Fortunately, however, there are pockets of organizational open-mindedness. Consider a major new research project funded by the Intelligence Advanced Research Projects Activity, a branch of the intelligence community.
In an unprecedented “forecasting tournament,” five teams will compete to see who can most accurately predict future political and economic developments. One of the five is Tetlock’s “Good Judgment” Team, which will measure individual differences in thinking styles among 2,400 volunteers (e.g., fox versus hedgehog) and then assign volunteers to experimental conditions designed to encourage alternative problem-solving approaches to forecasting problems. The volunteers will then make individual forecasts which statisticians will aggregate in various ways in pursuit of optimal combinations of perspectives. It’s hoped that combining superior styles of thinking with the famous “wisdom of crowds” will significantly boost forecast accuracy beyond the untutored control groups of forecasters who are left to fend for themselves.
Other teams will use different methods, including prediction markets and Bayesian networks, but all the results will be directly comparable, and so, with a little luck, we will learn more about which methods work better and under what conditions. This sort of research holds out the promise of improving our ability to peer into the future.
But only to some extent, unfortunately. Natural science has discovered in the past half-century that the dream of ever-growing predictive mastery of a deterministic universe may well be just that, a dream. There increasingly appear to be fundamental limits to what we can ever hope to predict. Take the earthquake in Japan. Once upon a time, scientists were confident that as their understanding of geology advanced, so would their ability to predict such disasters. No longer. As with so many natural phenomena, earthquakes are the product of what scientists call “complex systems,” or systems which are more than the sum of their parts. Complex systems are often stable not because there is nothing going on within them but because they contain many dynamic forces pushing against each other in just the right combination to keep everything in place. The stability produced by these interlocking forces can often withstand shocks but even a tiny change in some internal conditional at just the right spot and just the right moment can throw off the internal forces just enough to destabilize the system—and the ground beneath our feet that has been so stable for so long suddenly buckles and heaves in the violent spasm we call an earthquake. Barring new insights that shatter existing paradigms, it will forever be impossible to make time-and-place predictions in such complex systems. The best we can hope to do is get a sense of the probabilities involved. And even that is a tall order.
Human systems like economies are complex systems, with all that entails. And bear in mind that human systems are not made of sand, rock, snowflakes, and the other stuff that behaves so unpredictably in natural systems. They’re made of people: self-aware beings who see, think, talk, and attempt to predict each other’s behavior—and who are continually adapting to each other’s efforts to predict each other’s behavior, adding layer after layer of new calculations and new complexity. All this adds new barriers to accurate prediction.
When governments the world over were surprised by this year’s events in the Middle East, accusing fingers were pointed at intelligence agencies. Why hadn’t they seen it coming? “We are not clairvoyant,” James R. Clapper Jr, director of national intelligence, told a hearing of the House intelligence committee. Analysts were well aware that forces capable of generating unrest were present in Tunisia, Egypt, and elsewhere. They said so often. But those forces had been present for years, even decades. “Specific triggers for how and when instability would lead to the collapse of various regimes cannot always be known or predicted,” Clapper said.
That is a considerable understatement. Remember that it was a single suicidal protest by a lone Tunisian fruit seller that set off the tumult, just as an infinitesimal shift can apparently precipitate an earthquake. But even after the unrest had begun, predicting what would follow and how it would conclude was a fool’s errand because events were contingent on the choices of millions of people, and those choices were contingent on perceptions that could and did change constantly. Say you’re an Egyptian. You’re in Cairo. You want to go to the protest but you’re afraid. If you go and others don’t, the protest will fail. You may be arrested and tortured. But if everyone goes, you will have safety in numbers and be much likelier to win the day. Perhaps. It’s also possible that a massive turnout will make the government desperate enough to order soldiers to open fire. Which the soldiers may or may not do, depending in part on whether they perceive the government or the protestors to have the upper hand. In this atmosphere, rumors and emotions surge through the population like electric charges. Excitement gives way to terror in an instant. Despair to hope. And back again. What will people do? How will the government react? Nothing is certain until it happens. And then many pundits declare whatever happened was inevitable. Indeed, they saw it coming all along, or so they believe in hindsight.
So we are not blind but there are serious limits to how far we can see. Weather forecasting is a useful model to keep in mind. We joke about weather forecasters but they have some good mental habits we should all practice: making explicit predictions and revising them in response to clear timely feedback. The net result is that weather forecasters are one of the best calibrated of all professional groups studied—up there with professional bridge players. They have a good sense for what they do and do not know.
But well calibrated does not mean omniscient. As weather forecasters well know, their accuracy extends out only a few days. Three or four days out, they are less accurate. Beyond a week, you might as well flip a coin. As scientists learn more about weather, and computing power and sophistication grow, this forecasting horizon may be pushed out somewhat, but there will always be a point beyond which meteorologists cannot see, even in theory.
We call this phenomenon the diminishing marginal predictive returns of knowledge.
In political and economic forecasting, we reach the inflection point surprisingly quickly. It lies in the vicinity of attentive readers of high-quality news outlets, such as The Economist. The predictive value added of Ph.Ds, tenured professorships and Nobel Prizes is not zero but it is disconcertingly close to zero.
So we should be suspicious of pundits waving credentials and adopt the old trust-but-verify mantra: test the accuracy of forecasts and continually be on the lookout for new methods that improve results. We must also accept that even if we were to do this on a grand scale, and our forecasts were to become as accurate as we can possibly make them, there would still be failure, uncertainty, and surprise. And The World In Whatever-The-Next-Year-Is would continue to look quite different from the world in whatever the next year is.
It follows that we also need to give greater consideration to living with failure, uncertainty, and surprise.
Designing for resiliency is essential, as New Zealanders discovered in February when a major earthquake struck Christchurch. 181 people were killed. When a somewhat larger earthquake struck Haiti in 2010, it killed hundreds of thousands. The difference? New Zealand’s infrastructure was designed and constructed to withstand an earthquake, whenever it might come. Haiti’s wasn’t.
Earthquakes are among the least surprising surprises, however. The bigger test is the truly unexpected shock. That’s when the capacity to respond is critical, as Canada demonstrated following the financial meltdown of 2008. For a decade prior to 2008, Canada’s federal government ran budgetary surpluses and used much of that money to pay down accumulated debt. When the disaster struck, the economy tipped into recession, and the government responded with an array of expensive policies. The budget went into deficit, and the debt-to-GDP ratio rose, but by both measures Canada continued to be in far better shape than most other developed countries. If further shocks come in the immediate future, Canada has plenty of capacity to respond—unlike the United States and the many other countries that did not spend a decade strengthening their fiscal foundations.
Accepting that our foresight will always be myopic also calls for decentralized decision-making and a proliferation of small-scale experimentation. Test the way forward, gingerly, one cautious step at a time. “Cross the river by feeling for the stones,” as the wily Deng Xiaoping famously said about China’s economic liberalization. Only madmen are sure they know what the future holds; only madmen take great leaps forward.
There’s nothing terribly controversial in this advice. Indeed, it’s standard stuff in any discussion of forecasting and uncertainty. But critical caveats are seldom mentioned.
There’s the matter of marginal returns, for one. As with most things in life, the first steps in improving forecasting are the easiest and cheapest. It doesn’t take a lot of analysis to realize that goats’ entrails and tea leaves do a very poor job of weather forecasting, and it takes only a little more analysis to discover that meteorologists’ forecasts are much better, and that switching from the former to the latter makes sense even though the latter costs more than the former. But as we make further advances in weather forecasting, we are likely to find that each incremental improvement will be harder than the last, delivering less benefit at greater cost. So when do we say that further advances aren’t worth it?
The same is true of resiliency. Tokyo skyscrapers are built to the highest standards of earthquake resistance because it is close to certain that in their lifespan they will be tested by a major earthquake. Other skyscrapers in other cities not so prone to earthquakes could be built to the same standards but that would raise the cost of construction substantially. Is that worth doing? And if we accept a lower standard, how high is enough? And what about all the other low-probability, high-impact events that could strike? We could spend a few trillion dollars building a string of orbital defences against killer asteroids. If that seems like a waste, what about the few hundred million dollars it would take to spot and track most asteroids? That may seem like a more reasonable proposition, but remember that some asteroids are likely to escape our notice. Not to mention comets. Or the many other shocks the universe could conceivably hurl at us. There’s no limit to what we can spend preparing for unpleasant surprises, so how much is enough?
And notice what we have to do the moment we try to answer a question like, “is it worth constructing this skyscraper so it is more resistant to major earthquakes?” The answer depends on many factors but the most important is the likelihood that the skyscraper will ever have to resist a major earthquake. Happily, we’re good at determining earthquake probabilities. Less happily, we’re far from perfect. One reason why the Japanese disaster was so devastating is that an earthquake of such magnitude wasn’t expected where it occurred. Even less happily, we’re far better at determining earthquake probabilities than countless other important phenomena we want and need to forecast. Energy supplies. Recessions. Revolutions. There’s a very long list of important matters about which we really have no choice but to make probability judgements even though the evidence suggests our methods aren’t working a whole lot better than goats’ entrails and tea leaves.
The optimist thinks that’s fabulous because it means there’s lots of room for improvement. The pessimist stockpiles dry goods and ammunition. They both have a point.
The optimists are right that there is much we can do at a cost that is quite modest relative to what is often at stake. For example, why not build on the IARPA tournament? Imagine a system for recording and judging forecasts. Imagine running tallies of forecasters’ accuracy rates. Imagine advocates on either side of a policy debate specifying in advance precisely what outcomes their desired approach is expected to produce, the evidence that will settle whether it has done so, and the conditions under which participants would agree to say “I was wrong.” Imagine pundits being held to account. Of course arbitration only works if the arbiter is universally respected and it would be an enormous challenge to create an analytical center whose judgments were not only fair, but perceived to be fair even by partisans dead sure they are right and the other guys are wrong. But think of the potential of such a system to improve the signal-to-noise ratio, to sharpen public debate, to shift attention from blowhards to experts worthy of an audience, and to improve public policy. At a minimum, it would highlight how often our forecasts and expectations fail, and if that were to deflate the bloated confidence of experts and leaders, and give pause to those preparing some “great leap forward,” it would be money well spent.
But the pessimists are right, too, that fallibility, error, and tragedy are permanent conditions of our existence. Humility is in order, or, as Socrates said, the beginning of wisdom is the admission of ignorance. The Socratic message has always been a hard sell, and it still is—especially among practical people in business and politics, who expect every presentation to end with a single slide consisting of five bullet points labeled “The Solution.”
We have no such slide, unfortunately. But in defense of Socrates, humility is the foundation of the fox style of thinking and much research suggests it is an essential component of good judgment in our uncertain world. It is practical. Over the long term, it yields better calibrated probability judgments, which should help you affix more realistic odds than your competitors on policy bets panning out.
Humble works. Or it is at least superior to the alternative.
Response Essays
Who Cares About Forecast Accuracy?
Gardner and Tetlock note that while prediction is hard, we should be able to do better. For example, we could attend less to “hedgehogs” who know “one big thing” and whose forecasts are “beaten rather soundly even by the [random] chimp.” Yet we seem surprisingly uninterested in improving our forecasts:
Corporations and governments spend staggering amounts of money on forecasting, and one might think they would be keenly interested in determining the worth of their purchases and ensuring they are the very best available. But most aren’t. They spend little or nothing analyzing the accuracy of forecasts and not much more on research to develop and compare forecasting methods. Some even persist in using forecasts that are manifestly unreliable. … This widespread lack of curiosity … is a phenomenon worthy of investigation.
I can confirm that this disinterest is real. For example, when I try to sell firms on internal prediction markets wherein employees forecast things like sales and project completion dates, such firms usually don’t doubt my claims that such forecasts are cheap and more accurate. Nevertheless, they usually aren’t interested.
TV weather forecasters are not usually chosen based on their forecast accuracy. Top business professors tell me that firms usually aren’t interested in doing randomized experiments to test their predictions about business methods. Furthermore, a well-connected reporter told me that a major DC-area media firm recently abandoned a large project to collect pundit prediction track records, supposedly because readers just aren’t interested.
Gardner and Tetlock are heartened to see a big research project testing new ways to aggregate forecasts, as that “holds out the promise of improving our ability to peer into the future.” But I can’t be much encouraged without a better understanding of our disinterest in using already well-tested and simpler methods. After all, a good diagnosis usually precedes a good prognosis.
So let me try to tackle this puzzle head on. Surprising disinterest in forecasting accuracy could be explained either by its costs being higher, or its benefits being lower, than we expect.
The costs of creating and monitoring forecast accuracy might be higher than we expect if in general thinking about times other than the present is harder than we expect. Most animals seem to focus almost entirely on reacting to current stimuli, as opposed to remembering the past or anticipating the future. We humans are proud that we attend more to the past and future, but perhaps this is still harder than we let on, and we flatter ourselves by thinking we attend more than we do.
The benefits of creating and monitoring forecast accuracy might be lower than we expect if the function and role of forecasting is less important than we think, relative to the many functions and roles served by our pundits, academics, and managers.
Consider first the many possible functions and roles of media pundits. Media consumers can be educated and entertained by clever, witty, but accessible commentary, and can coordinate to signal that they are smart and well-read by quoting and discussing the words of the same few focal pundits. Also, impressive pundits with prestigious credentials and clear “philosophical” positions can let readers and viewers gain by affiliation with such impressiveness, credentials, and positions. Being easier to understand and classify helps “hedgehogs” to serve many of these functions.
Second, consider the many functions and roles of academics. Academics are primarily selected and rewarded for their impressive mastery and application of difficult academic tools and methods. Students, patrons, and media contacts can gain by affiliation with credentialed academic impressiveness. In forecasts, academic are rewarded much more for showing mastery of impressive tools than for accuracy.
Finally, consider next the many functions and roles of managers, both public and private. By being personally impressive, and by being identified with attractive philosophical positions, leaders can inspire people to work for and affiliate with their organizations. Such support can be threatened by clear tracking of leader forecasts, if that questions leader impressiveness.
Even in business, champions need to assemble supporting political coalitions to create and sustain large projects. As such coalitions are not lightly disbanded, they are reluctant to allow last minute forecast changes to threaten project support. It is often more important to assemble crowds of supporting “yes-men” to signal sufficient support, than it is to get accurate feedback and updates on project success. Also, since project failures are often followed by a search for scapegoats, project managers are reluctant to allow the creation of records showing that respected sources seriously questioned their project.
Often, managers can increase project effort by getting participants to see an intermediate chance of the project making important deadlines—the project is both likely to succeed, and to fail. Accurate estimates of the chances of making deadlines can undermine this impression management. Similarly, overconfident managers who promise more than they can deliver are often preferred, as they push teams harder when they fall behind and deliver more overall.
Even if disinterest in forecast accuracy is explained by forecasting being only a minor role for pundits, academics, and managers, might we still hope for reforms to encourage more accuracy? If there is hope, I think it mainly comes from the fact that we pretend to care more about forecast accuracy than we actually seem to care. We don’t need new forecasting methods so much as a new social equilibrium, one that makes forecast hypocrisy more visible to a wider audience, and so shames people into avoiding such hypocrisy.
Consider two analogies. First, there are cultures where few requests made of acquaintances are denied. Since it is rude to say “no” to a difficult request, people instead say “yes,” but then don’t actually deliver. In other cultures, it is worse to say “yes” but not deliver, because observers remember and think less of those who don’t deliver. The difference is less in the technology of remembering, and more in the social treatment of such memories.
A second analogy is that in some cultures people who schedule to meet at particular times actually show up over a wide range of surrounding times. While this might once have been reasonable given uncertain travel times and unreliable clocks, such practices continued long after these problems were solved. In other cultures, people show up close to scheduled meeting times, because observers remember and think less of those who are late.
In both of these cases, it isn’t enough to just have a way to remember behavior. A track record tech must be combined with a social equilibrium that punishes those with poor records, and thus encourages rivals and victims to collect and report records. The lesson I take for forecast accuracy is that it isn’t enough to devise ways to record forecast accuracy—we also need a new matching social respect for such records.
Might governments encourage a switch to more respect for forecast accuracy? Yes: by not explicitly discouraging it! Today, the simplest way to create forecast track records that get attention and respect is by making bets. In a bet, the parties work to define the disputed issue clearly enough to resolve later, and the bet payoff creates a clear record of who was right and wrong. Anti-gambling laws now discourage such bets—shouldn’t we at least eliminate this impediment to more respect for forecast accuracy records?
And once bets are legal we should go further, to revive our ancestors’ culture of respect for bets. It should be shameful to visibly disagree and yet evade a challenge to more clearly define the disagreement and bet a respectable amount on it. Why not let “put your money where your mouth is” be our forecast-accuracy-respecting motto?
In Defense of the Hedgehogs
It is true, as Dan Gardner and Philip Tetlock point out, that economic forecasting isn’t very good. Financial forecasting is next to useless. At least these are better than political forecasting, and at least economic and financial forecasters routinely use statistical models, compare judgmental and statistical forecasts with outcomes, and systematically improve. (I refer to real forecasters here, not the clowns on TV.) But many movements of the economy and financial markets are so far beyond anyone’s ability to foresee.
Unforecastability Is a Good Sign
It is also true, as they hint, that the reason for this is the inherent unforecastability of the system, not the incompetence of the forecasters. One should not conclude from “you didn’t forecast the crash” that “economists don’t know what they’re doing,” or “the economy is all screwed up and needs lots of regulating.”
In fact, many economic events should be unforecastable, and their unforecastability is a sign that the markets and our theories about them are working well.
This statement is clearest in the case of financial markets. If anyone could tell you with any sort of certainty that “the market will go up tomorrow,” you could use that information to buy today and make a fortune. So could everyone else. As we all try to buy, the market would go up today, right to the point that nobody can tell whether tomorrow’s value will be higher or lower.
An “efficient” market should be unpredictable. If markets went steadily up and delivered return without risk, then markets would not be working as they should.
Much the same happens throughout economics. Consumption should depend on “permanent” income, as Milton Friedman pointed out. That means today’s consumption should depend on consumers’ best guess of future prospects, just as a stock price is investors’ best guess of future returns. Changes in consumption, driven by changes in information, therefore should be just as unpredictable as stock prices.
Economics often predicts unpredictability even when markets are not working well. A bank run is an undesirable outcome, but the theory of bank runs says they should be unpredictable. If anyone knew the run would happen tomorrow, it would happen today.
Gardner and Tetlock cite complex systems and nonlinear dynamics, but even these mathematical structures have been failures in forecasting economic and financial systems. Complex and nonlinear dynamic systems are predictable, they are just very sensitive to initial conditions. Tests for nonlinearities in the sciences found them popping up all over. Except in the stock market. The fact that we who study the system are part of the system, that people can read our papers and forecasts, and change their behavior as a result, means that we are no smarter than the system we study. Indeed, this makes the domain of social sciences uniquely unforecastable.
Some trends in economics are nonetheless predictable. When things get out of whack you can tell they will converge. Unemployment of 9% won’t last forever (unless the government really screws things up); a huge debt to GDP ratio must be resolved by growth, default, or inflation. If you take a billion people, terrorize them to the stone age, and then get out of the way a bit, their wealth and incomes will grow very fast for a while as they catch up (China). But even here, the slow movement of predictable long-run trends is swamped by shorter-run unpredictable variation.
Risk Management Rather than Forecast-and-Plan
The answer is to change the question, to focus on risk management, as Gardner and Tetlock suggest. There is a set of events that could happen tomorrow—Chicago could have an earthquake, there could be a run on Greek debt, the Administration could decide “Heavens, Dodd–Frank and Obamacare were huge mistakes, let’s fix them” (Okay, not the last one.) Attached to each event, there is some probability that it could happen.
Now “forecasting” as Gardner and Tetlock characterize it, is an attempt to figure out which event really will happen, whether the coin will land on heads or tails, and then make a plan based on that knowledge. It’s a fool’s game.
Once we recognize that uncertainty will always remain, risk management rather than forecasting is much wiser. Just the step of naming the events that could happen is useful. Then, ask yourself, “if this event happens, let’s make sure we have a contingency plan so we’re not really screwed.” Suppose you’re counting on diesel generators to keep cooling water flowing through a reactor. What if someone forgets to fill the tank?
The good use of “forecasting” is to get a better handle on probabilities, so we focus our risk management resources on the most important events. But we must still pay attention to events, and buy insurance against them, based as much on the painfulness of the event as on its probability. (Note to economics techies: what matters is the risk-neutral probability, probability weighted by marginal utility.)
So it’s not really the forecast that’s wrong, it’s what people do with it. If we all understood the essential unpredictability of the world, especially of rare and very costly events, if we got rid of the habit of mind that asks for a forecast and then makes “plans” as if that were the only state of the world that could occur; if we instead focused on laying out all the bad things that could happen and made sure we had insurance or contingency plans, both personal and public policies might be a lot better.
Foxes and Hedgehogs
Gardner and Tetlock admire the “foxes” who “used a wide assortment of analytical tools, sought out information from diverse sources, were comfortable with complexity and uncertainty, and were much less sure of themselves… they frequently shifted intellectual gears.” By contrast, “hedgehogs” “tended to use one analytical tool in many different domains, … preferred keeping their analysis simple and elegant by minimizing “distractions” and zeroing in on only essential information.”
There is another very important kind of “forecast” however, and here I think some “hedgehog” traits have an advantage.
Gardner and Tetlock have in mind what economists call “unconditional” forecasting. In this, they are content to use historical correlations to guess what comes next, with no need of structural understanding. We often do this in economic forecasting, and rightly. For example, the slope of the yield curve gives a good signal of whether recessions are coming. But this does not mean that if the government changes that slope it will change the recession. Forcing the weather forecaster to lie will not produce a sunny weekend. Leading indicators, confidence surveys, and more formal regression-based and statistical forecasts all operate this way.
But economics is really concerned with conditional forecasting; predicting the answers to questions such as “if we pass a trillion dollar stimulus, how much more GDP will we get next year?” “If we raise taxes on ‘the rich’, how much less will they work, and how much revenue will we actually raise?” “If the Fed monetizes $600 billion of long-term debt, how much will GDP increase, and much inflation will we get, and how soon?” “If you tell insurance companies they have to take everyone at the same price no matter how sick, how many will sign up for insurance?”
Here we are trying to “predict” the effect of a policy, how much the future will change if a policy is enacted. Despite popular impression, the vast majority of economists spend the vast majority of their time on these sorts of questions, not on unconditional forecasts. Asking the average economist whether unemployment will go down next quarter is about as useless as asking a meteorological researcher who studies the physics of tornadoes whether it will rain over the weekend. He probably doesn’t even have a window in his office.
It was once hoped that really understanding the structure of the economy would also help in the sort of unconditional forecasting that Gardner and Tetlock are more interested in. Alas, that turned out not to be true. Big “structural” macroeconomic models predict no better than simple correlations. Even if you understand many structural linkages from policy to events, there are so many other unpredictable shocks that imposing “structure” just doesn’t help with unconditional forecasting.
But economics can be pretty good at such structural forecasting. We really do know what happens if you put in minimum wages, taxes, tariffs, and so on. We have a lot of experience with regulatory capture. At least we know the signs and general effects. Assigning numbers is a lot harder. But those are useful predictions, even if they typically dash youthful liberal hopes and dreams.
Doing good forecasting of this sort, however, rewards some very hedgehoggy traits.
Focusing on “one analytical tool”—basic supply and demand, a nose for free markets, unintended consequences, and regulatory capture—is essential. People who use a wide range of analytical tools, mixing economics, political, sociological, psychological, Marxist-radical and other perspectives end up hopelessly muddled.
Keeping analysis “simple and elegant” and “minimizing distractions” is vital too, rather than being “comfortable with complexity and uncertainty,” or even being “much less sure of oneself.” Especially around policy debates, one is quickly drowned in mind-blowing detail. Keeping the simple picture and a few basic principles in mind is the only hope.
Gardner and Tetlock admire statistical modeling, but this is usually a smokescreen in conditional forecasting, and only serves to hide the central stories about which we actually know something.
Milton Friedman was a hedgehog. And he got the big picture of cause and effect right in a way that the foxes around him completely missed. Take just one example, his 1968 American Economic Association presidential speech, in which he said that continued inflation would not bring unemployment down, but would lead to stagflation. He used simple, compelling logic, from one intellectual foundation. He ignored big computer models, statistical correlations, and all the muddle around him. And he was right.
In political forecasting, anyone’s success in predicting cause and effect is even lower. U.S. foreign policy is littered with cause-and-effect predictions and failures—if we give them money, they’ll love us; if we invade they will welcome us as liberators; if we pay both sides they will work for peace, not keep the war and subsidies going forever.
But the few who get it right are hedgehogs. Ronald Reagan was a hedgehog, sticking to a few core principles that proved to be right.
Good hedgehogs are not know-it-alls. Friedman didn’t produce a quarterly inflation forecast, and he argued against all the “fine tuning” in which the Fed indulges to this day. Good hedgehogs stick to a few core principles because they know that nobody really knows detailed answers.
Principles matter. They produce wiser conditional forecasts. That’s a good thing for this forum, because otherwise the Cato Institute should disband!
Fox-Hedging or Knowing: One Big Way to Know Many Things
It is hard to say which is more surprising, that anyone still argues that we can predict very little or that anyone believes expertise conveys reliable judgment. Each reflects a bad habit of mind that we should overcome. It is certainly true that predictive efforts, by whatever means, are far from perfect and so we can always come up with examples of failure. But a proper assessment of progress in predictive accuracy, as Gardner and Tetlock surely agree, requires that we compare the rate of success and failure across methods of prediction rather than picking only examples of failure (or success). How often, for instance, has The Economist been wrong or right in its annual forecasts compared to other forecasters? Knowing that they did poorly in 2011 or that they did well in some other selected year doesn’t help answer that question. That is why, as Gardner and Tetlock emphasize, predictive methods can best be evaluated through comparative tournaments.
Reliable prediction is so much a part of our daily lives that we don’t even notice it. Consider the insurance industry. At least since Johan de Witt (1625–1672) exploited the mathematics of probability and uncertainty, insurance companies have generally been profitable. Similarly, polling and other statistical methods for predicting elections are sufficiently accurate most of the time that we forget that these methods supplanted expert judgment decades ago. Models have replaced pundits as the means by which elections are predicted exactly because various (imperfect) statistical approaches routinely outperform expert prognostications. More recently, sophisticated game theory models have proven sufficiently predictive that they have become a mainstay of high-stakes government and business auctions such as bandwidth auctions. Game theory models have also found extensive use and well-documented predictive success on both sides of the Atlantic in helping to resolve major national security issues, labor-management disputes, and complex business problems. Are these methods perfect or omniscient? Certainly not! Are the marginal returns to knowledge over naïve methods (expert opinion; predicting that tomorrow will be just like today) substantial? I believe the evidence warrants an enthusiastic “Yes!” Nevertheless, despite the numerous successes in designing predictive methods, we appropriately focus on failures. After all, by studying failure methodically we are likely to make progress in eliminating some errors in the future.
Experts are an easy, although eminently justified, target for critiquing predictive accuracy. Their failure to outperform simple statistical algorithms should come as no surprise. Expertise has nothing to do with judgment or foresight. What makes an expert is the accumulation of an exceptional quantity of facts about some place or time. The idea that such expertise translates into reliable judgment rests on the false belief that knowing “the facts” is all that is necessary to draw correct inferences. This is but one form of the erroneous linkage of correlation to causation; a linkage at the heart of current data mining methods. It is even more so an example of confusing data (the facts) with a method for drawing inferences. Reliance on expert judgment ignores their personal beliefs as a noisy filter applied to the selection and utilization of facts. Consider, for instance, that Republicans, Democrats, and libertarians all know the same essential facts about the U.S. economy and all probably desire the same outcomes: low unemployment, low inflation, and high growth. The facts, however, do not lead experts to the same judgment about what to do to achieve the desired outcomes. That requires a theory and balanced evidence about what gets us from a distressed economy to a well-functioning one. Of course, lacking a common theory and biased by personal beliefs, the experts’ predictions will be widely scattered.
Good prediction—and this is my belief—comes from dependence on logic and evidence to draw inferences about the causal path from facts to outcomes. Unfortunately, government, business, and the media assume that expertise—knowing the history, culture, mores, and language of a place, for instance—is sufficient to anticipate the unfolding of events. Indeed, too often many of us dismiss approaches to prediction that require knowledge of statistical methods, mathematics, and systematic research design. We seem to prefer “wisdom” over science, even though the evidence shows that the application of the scientific method, with all of its demands, outperforms experts (remember Johan de Witt). The belief that area expertise, for instance, is sufficient to anticipate the future is, as Tetlock convincingly demonstrated, just plain false. If we hope to build reliable predictions about human behavior, whether in China, Cameroon, or Connecticut, then probably we must first harness facts to the systematic, repeated, transparent application of the same logic across connected families of problems. By doing so we can test alternative ways of thinking to uncover what works and what doesn’t in different circumstances. Here Gardner, Tetlock, and I could not agree more. Prediction tournaments are an essential ingredient to work out what the current limits are to improved knowledge and predictive accuracy. Of course, improvements in knowledge and accuracy will always be a moving target because technology, ideas, and subject adaptation will be ongoing.
Given what we know today and given the problems inherent in dealing with human interaction, what is a leading contender for making accurate, discriminating, useful predictions of complex human decisions? In good hedgehog mode I believe one top contender is applied game theory. Of course there are others but I am betting on game theory as the right place to invest effort. Why? Because game theory is the only method of which I am aware that explicitly compels us to address human adaptability. Gardner and Tetlock rightly note that people are “self-aware beings who see, think, talk, and attempt to predict each other’s behavior—and who are continually adapting to each other’s efforts to predict each other’s behavior, adding layer after layer of new calculations and new complexity.” This adaptation is what game theory jargon succinctly calls “endogenous choice.” Predicting human behavior means solving for endogenous choices while assessing uncertainty. It certainly isn’t easy but, as the example of bandwidth auctions helps clarify, game theorists are solving for human adaptability and uncertainty with some success. Indeed, I used game theoretic reasoning on May 5, 2010 to predict to a large investment group’s portfolio committee that Mubarak’s regime faced replacement, especially by the Muslim Brotherhood, in the coming year. That prediction did not rely on in-depth knowledge of Egyptian history and culture or on expert judgment but rather on a game theory model called selectorate theory and its implications for the concurrent occurrence of logically derived revolutionary triggers. Thus, while the desire for revolution had been present in Egypt (and elsewhere) for many years, logic suggested that the odds of success and the expected rewards for revolution were rising swiftly in 2010 in Egypt while the expected costs were not.
This is but one example that highlights what Nobel laureate Kenneth Arrow, who was quoted by Gardner and Tetlock, has said about game theory and prediction (referring, as it happens, to a specific model I developed for predicting policy decisions): “Bueno de Mesquita has demonstrated the power of using game theory and related assumptions of rational and self-seeking behavior in predicting the outcome of important political and legal processes.” Nice as his statement is for me personally, the broader point is that game theory in the hands of much better game theorists than I am has the potential to transform our ability to anticipate the consequences of alternative choices in many aspects of human interaction.
How can game theory be harnessed to achieve reliable prediction? Acting like a fox, I gather information from a wide variety of experts. They are asked only for specific current information (Who wants to influence a decision? What outcome do they currently advocate? How focused are they on the issue compared to other questions on their plate? How flexible are they about getting the outcome they advocate? And how much clout could they exert?). They are not asked to make judgments about what will happen. Then, acting as a hedgehog, I use that information as data with which to seed a dynamic applied game theory model. The model’s logic then produces not only specific predictions about the issues in question, but also a probability distribution around the predictions. The predictions are detailed and nuanced. They address not only what outcome is likely to arise, but also how each “player” will act, how they are likely to relate to other players over time, what they believe about each other, and much more. Methods like this are credited by the CIA, academic specialists and others, as being accurate about 90 percent of the time based on large-sample assessments. These methods have been subjected to peer review with predictions published well ahead of the outcome being known and with the issues forecast being important questions of their time with much controversy over how they were expected to be resolved. This is not so much a testament to any insight I may have had but rather to the virtue of combining the focus of the hedgehog with the breadth of the fox. When facts are harnessed by logic and evaluated through replicable tests of evidence, we progress toward better prediction.
We can all hope that government, academia, and the media will rally behind Gardner and Tetlock’s pursuit of systematic tests of alternative methods for predicting the future. Methodical tournaments of alternative methods surely will go a long way to advancing our understanding of how logic and evidence can convert mysteries into the known and knowable.
The Conversation
Designing Fair Tests for the Hedeghogs
Over decades, Philip Tetlock painstakingly collected data on simple long-term forecasts in political economy. He showed that hedgehogs, who focus on one main analytical tool, are less accurate than foxes, who used a wide assortment of analytical tools. Since John Cochrane and Bruce Bueno De Mesquita are both prototypical hedgehogs, I was curious to see their response to Tetlock’s data.
Cochrane argues that no one can do well at the unconditional forecasts that Tetlock studied, and that forecasting is less important than people presume. But he says that hedgehogs shine at important conditional forecasts, such as GDP change given a big stimulus, or the added tax revenue given higher tax levels.
De Mesquita says that while “government, business, and the media” prefer “experts” who know many specific facts, such as “the history, culture, mores, and language of a place,” “scientists” who know “statistical methods, mathematics, and systematic research design” are more accurate. He also notes that his hedgoggy use of game theory is liked by the CIA and by peer review.
Of course even before Tetlock’s study we knew that both peer review and funding patrons bestowed plenty of approval on hedgehogs, who usually claim that they add important forecasting value. Tetlock’s new contribution is to use hard data to question such claims. Yes, Tetlock’s data is hardly universal, so that leaves room for counter-claims about missing important ways in which hedgehogs are more accurate. But I find it disappointing, and also a bit suspicious, that neither Cochrane nor De Mesquita express interest in helping to design better studies, much less in participating in such studies.
De Mesquita is proud that his methods seem to achieve substantial accuracy, but he has not to my knowledge participated in open competitions giving a wide range of foxes a chance to show they could achieve comparable accuracy. Yes, academic journals are “open competitions,” but the competition is not on the basis of forecast accuracy.
Regarding Cochrane’s conditional accuracy claims, it is certainly possible to collect and score accuracy on conditional forecasts. One need only look at the conditions that turned out to be true, and score the forecasts for those conditions. The main problem is that this approach requires more conditional forecasts to get the same statistical power in distinguishing forecast accuracy. Since Tetlock’s study was a monumental effort, a similar study with conditional forecasts would be even more monumental. Would Cochrane volunteer for such a study?
I expect that, like most academics, both Cochrane and De Mesquita would demand high prices to publicly participate in an accuracy-scored forecasting competition in which foxes could also compete. Remember that Tetlock had to promise his experts anonymity to get them to participate in his study. The sad fact is that the many research patrons eager to fund hedgehoggy research by folks like Cochrane and De Mesquita show little interest in funding forecasting competitions at the scale required to get public participation by such prestigious folks. So hedgehogs like Cochrane and De Mesquita can continue to claim superior accuracy, with little fear of being proven wrong anytime soon.
All of which brings us back to our puzzling disinterest in forecast accuracy, which was the subject of my response to the Gardner and Tetlock essay. I still suspect that most who pay to affiliate with prestigious academics care little about their accuracy, though they do like to claim to care.
The Tests Are Underway
Robin Hanson’s comments intrigue me. He seems to believe that I am reluctant to subject my method to comparison to alternative methods and that I have not been open to doing so in the past. Nothing could be further from the truth. He does correctly note that academic journals are an open competition but, as he says, “the competition is not on the basis of forecast accuracy.” Well, that is true and not true.
Journal acceptance is presumably based on the quality of the work, including its added-value. Of course, it cannot be based on the accuracy of any forecasts contained in a paper under review since the point of forecasting is to address questions whose answers are not yet known. But here is where Hanson goes astray. As I argued in chapter 10 of The Predictioneer’s Game and in other publications as well (going back as far as the 1980s), researchers should dare to be embarrassed by putting their predictions in print before outcomes are known. Thus, it seems that the “competitors” who have not done so are the ones reluctant to face competition and not me. Peer review is a vehicle for daring to be embarrassed. I have an observable track record in journal publications open to scrutiny regarding accuracy; the bulk of “competitors” seem reluctant to subject themselves to just such evaluation. I urge them to do so.
Hanson is mistaken about me in other important ways. Contrary to the reluctance attributed to me, I and others have several publications testing alternative models against the same data in an open competition of ideas. This includes, for instance, the 1994 volume European Community Decision Making: Model, Applications and Comparisons and the 2006 volume Europe Decides; as well as articles with prominent prospect theorists in peer-reviewed journals, and numerous other published studies. Additionally, I participated in a competition organized by the Defense Threat Reduction Agency in 2003 in which approximately six different quantitative approaches to forecasting were applied to answering the same questions about al Qaeda tactics and targets over a two year window. It happens that my method “won” the competition while also being the least expensive project undertaken by the competitors. Finally, I have provided Philip Tetlock with dozens of studies by my undergraduate students who have enrolled in my course, Solving Foreign Crises, over the past several years in the hope that he will be able to evaluate their accuracy against alternative predictions within the confines of the IARPA project.
It is true that it can be costly to carry out predictions with my method. The data typically come from country or issue experts (who are asked for facts, not judgments) who must be paid for their time (my students manage to assemble data from information available on the web). But it is not true that the cost (in an academic setting) is large or that the model is not readily available for comparative testing. I have made my current model freely available online to bona fide faculty and students for course use or academic research use since 2009. My older model was made freely available online in association with my introductory international relations textbook starting around 1999. Hundreds of scholars and students have signed up for my current model and use it with some regularity. Several publications by others have already resulted from the use of that software. Anyone wishing to test other methods against mine for academic research purposes can register (it is free) to use the online software and conduct the very tests that Hanson calls for. That is why I have made the method available. So, yes I am proud of the accuracy achieved; I am eager for comparative testing; and will be more than happy if an alternative method proves better. That, after all, is how progress is made in the study of political decisionmaking.
Forecasting Tournaments Should Cast a Wider Net
I’m happy to learn that Bruce Bueno de Mesquita has made specific predictions in some of his publications, and has participated in some forecasting competitions. He deserves praise for being forthright in this way.
But the specific issue was the relative accuracy of foxes and hedgehogs, and my claim was about “open competitions giving a wide range of foxes a chance to show they could achieve comparable accuracy.” Tetlock’s study met this standard, but “a competition … in which approximately six different quantitative approaches to forecasting were applied” does not—mostly likely all were hedgehog approaches. “An observable track record in journal publications” also does not meet that standard, unless it is plausible that diverse foxes with access to acceptable publication forums could know sufficiently in advance on what topics and at what times to make their forecasts, in order to be comparable to De Mesquita’s.
An Unpredicted Outcome of Our Exchange: Testable Hypotheses
Each of the three commentaries on our target essay highlights obstacles to distinguishing real from pseudo-expertise in the political realm—and raises issues that we welcome the chance to address. Robin Hanson poses a puzzle: Why are thoughtful people so reluctant to embrace technologies, such as prediction markets, that have a track record of improving predictive accuracy? John Cochrane defends beleaguered hedgehogs by proposing that the comparative advantage of hedgehogs lies in their deep understanding of causal linkages and their ability to generate accurate conditional forecasts, something that we failed to capture in the forecasting exercises in Expert Political Judgment. And Bruce Bueno de Mesquita brings into focus the dangers of treating the fox and hedgehog approaches to knowledge as mutually exclusive. He suggests that hybrid approaches, such as his own, are best positioned to optimize forecasting performance in a noisy and densely interdependent world.
Readers hoping for polemical sparks to fly will be disappointed. To be sure, we have our disagreements with the commentators. But we see the arguments they raise as reasonable—and, far more important, testable. We would not be upset if subsequent work found support for each. And we see no reason why reasonable people cannot come to agreement on what would count as fair tests of these hypotheses. In a nutshell, we see wonderful opportunities to advance knowledge—and let the ideological chips fall where they may—which is the spirit of both the Expert Political Judgment project and its IARPA sequel.
Robin Hanson and Forecasting Tournaments: Threatening to Elites and Enticing to Their Challengers
Hanson’s reply brought to mind the scene in the movie A Few Good Men in which Jack Nicholson, the colonel in charge of Guantánamo, is badgered by a young idealistic attorney, Tom Cruise, in a murder investigation. In response to the attorney’s earnest entreaties that he just wants the “truth,” Nicholson launches into a tirade: the idealistic attorney has no inkling of the ugly trade-offs that the defense of liberty requires. He wouldn’t recognize, less tolerate, the truth if it stared him in the face.
That scene came to mind because Hanson finds so little real-world interest in the “truth.” Excellent econo-detective that he is, he explores the implications of this finding via deductive logic: (1) simple inexpensive tools, such as prediction markets, are already easily available for assessing accuracy and for improving it; (2) many people in business and government are aware of the efficacy of these tools but still do not embrace them; (3) so there must be either hidden costs to these tools beyond their nominal price tags or fewer benefits than advocates are supposing.
To appreciate these hidden costs, imagine that you are a renowned opinion guru, or possibly a lesser luminary in the same field. Multitudes (or just a few) read your commentaries. You are moderately famous and wealthy (or not). You may have a hard core of fans and a much larger group of quite regular readers who think you know what you’re talking about. You might be Tom Friedman or Paul Krugman or Holman Jenkins or David Brooks, or someone we’ve never heard of yet. How would you react if upstart academics—such as Robin Hanson or Phil Tetlock—pressed you to participate in a level-playing field exercise for assessing your relative forecasting accuracy: relative, that is, to rival pundits, relative to your readers, and relative to crude extrapolation algorithms or random-guess generators?
If you’re a big fish, you would be less than thrilled. What’s in it for you? If you perform poorly, you take a big reputation hit. Presumably your fans read your columns because they think they are getting a more accurate bead on where events are heading than they could obtain by spinning off predictions of their own or randomly throwing darts at a futures board—
—and they might be disappointed if disabused of that notion. And if you perform relatively well, you might take a hit anyway because you’re unlikely to do much better than your fans and quite likely to do a bit worse than simple extrapolation algorithms. You break even only in the extremely unlikely event that your forecasting performance matches the idealized image that your followers have of you. There just aren’t many six-sigma forecasters out there—by statistical definition. The likelihood of Tom Friedman delivering a performance that justifies his speaking fees is extremely low. The sensible thing is not to rock the boat: continue offering opinions that presuppose you must have deep insights into the future but at all cost never get pinned down.
The same logic applies to the smaller fish, whose opinions are not distributed daily to millions but may still carry cachet inside their companies. Open prediction contests will reveal how hard it is to outperform their junior assistants and secretaries. Insofar as technologies such as prediction markets make it easier to figure out who has better or worse performance over long stretches, prediction markets create exactly the sort of transparency that destabilizes status hierarchies. From this standpoint, it is impressive that Robin Hanson has gotten as wide a hearing as he has for his subversive agenda inside organizations—although that might be because elites must at least feign an interest in the truth.
If these hypotheses are correct the prognosis for prediction markets—and transparent competitions of relative forecasting performance—is grim. Epistemic elites are smart enough to recognize a serious threat to their dominance.
A powerful countervailing force needs to come into play to make the marketplace of ideas more vigorously competitive. But who or what should that be?
The IARPA forecasting exercise is a tentative step toward institutionalizing open prediction forums that allow testing the predictive power a wide range of individuals, techniques, and methods of blending individual and technical approaches (we count prediction markets as one of the most promising of the technologies). The more widely known and respected such competitions become, the harder it will be for pundits to continue business as usual—which means appearing to make bold claims about future states of the world but never really doing so (because their “predictions” are typically vague and cushioned from disconfirmation by complex conditionals, as we will discuss below.
One desirable result would be the emergence of a Consumer Reports for political-economic punditry that tracks the relative accuracy in different policy domains of representatives of clashing perspectives. But if wishes were horses, beggars would ride. The challenges will be finding sponsors ready to bankroll the operation (government sponsorship is obviously problematic—so volunteers please) and finding pundits ready to make clear claims that can be tested for accuracy (passing “the clairvoyance test”). These challenges are formidable but in our view the potential long-term benefits are large indeed. You don’t need to reduce the likelihood of multi-trillion-dollar mistakes by much to make a multi-million dollar investment pass the expected-value test.
John Cochrane in Defense of Hedgehogs
Cochrane rises to the defense of hedgehogs—our shorthand designation for the style of thinking associated with the worst predictive performance in the forecasting tournaments reported in Expert Political Judgment. Our treatment of the pros and cons of the different thinking styles—foxes versus hedgehogs—was somewhat cursory in our target article, so we would like to offer some reassurance to Cochrane, who might be surprised to discover that each of his defenses has already been given a hearing either in the 2005 book or in the 2010 fifth anniversary symposium in Critical Review. And he might be even more surprised to learn that we believe there is at least potential merit in each of the defenses that he raises.
Let’s start by noting that how easy it is a negative or positive political spin on both the fox and the hedgehog styles of reasoning. The negative spin—on which we admittedly focus in our target article—is that hedgehogs are both simpleminded and overconfident. They fall in love with one big idea and over apply that idea to a messy, exception-riddled empirical world. The positive spin on the hedgehog style of reasoning—which we largely ignored in our target article—is that hedgehogs can be bold visionaries who come up with incisive parsimonious explanations and solutions. The positive spin on foxes is that they are creative eclectics who managed to avoid the greatest blunders of hedgehogs and often succeed in crafting viable compromise policies. The negative spin on foxes is that they are confused, indecisive, and unprincipled. For the record, we believe there are good historical illustrations of each phenomenon.
But it is one thing to find examples and quite another to keep systematic store of relative accuracy. And here hedgehogs simply do not fare well—at least so far. And that is why we doubt Cochrane’s hypothesis that hedgehogs lost to foxes in the initial round of forecasting tournaments because we asked for crude unconditional rather than subtle conditional forecasts.
That said, Cochrane’s hypothesis still deserves to be systematically tested. Unfortunately, it is very hard to test.
Consider a prototypic conditional forecast: if policy x is adopted, expect outcome y (within this range of subjective probabilities) but if policy v is adopted, expect outcome w. The problem is that assessing the accuracy of conditional forecasts requires first assessing whether the conditionals were realized or implemented. That means that policy x or v—exactly as the forecaster intended it—was enacted. When something close to x or v was enacted but something not all that close to outcome y followed, the forecasters have a lot of wiggle room. They can always insist—as forecasters did in chapter 4 of Expert Political Judgment—that the conditionals underlying their forecasts were not satisfied.
Putting the enormous practical difficulty of the task to the side, we also have two empirical reasons for doubting Cochrane’s hypothesis: (a) there is virtually zero evidence in Expert Political Judgment, or anywhere else, that experts are any better or worse at predicting policy shifts within regimes, or changes in regimes (the most common antecedents of conditionals) than they are at predicting economic, geopolitical, or military outcomes (the most common consequences of the conditionals); (b) there is considerable evidence that, if anything, experts become decreasingly accurate in judging the probabilities of increasingly complex conjunctions of events (see the scenario-induced inflation of subjective probabilities in Chapter 7 of EPJ (and ‘‘the conjunction fallacy’’ in Tversky and Kahneman 1983). For instance, forecasters—sometimes even really smart ones—often perversely assign a higher probability to a plausible-sounding conjunction of events—such as a dam collapse in California causing mass drowning—than they do to the judged-less-likely linkage in the argument (mass drowning in California, with or without a dam collapse). Of course, this is logically impossible. The dam collapse is not the only path to mass drowning in California.
A more plausible defense of hedgehogs, in our view, is that relative to the foxes (in this context maybe better dubbed “chickens”), the hedgehogs are bold forecasters who account for a disproportionate number of grand slam homeruns. When something really surprising happens—a dramatic decline or expansion of economic growth or dramatic increases or decreases in international tension—there are usually hedgehogs who can claim credit for the predictions. Of course, there is no free lunch. The price of these occasional spectacular hits is a high rate of false positives, which hedgehogs must be willing to defend on the grounds that the “hits” are worth it. And that is a value judgment. Hedgehogs tend to be overrepresented at the extremes of opinion distributions—and to make more extreme forecasts that reflect their distinctive value priorities.
Bruce Bueno de Mesquita and the Predictive Power of Game Theory
Bruce Bueno de Mesquita’s reaction essay reframes hedgehog-fox distinction. He raises the plausible and quite testable hypothesis that the optimal approach to forecasting will prove over the long run to be one that combines the distinctive strengths of the more opportunistic foxes and the more theory-driven, deductive style of reasoning of hedgehogs. Bueno de Mesquita’s methodology arguably does this by drawing on the raw knowledge of area studies experts (whom he thinks are more likely to be “foxes”) and then integrating this knowledge using basic principles of decision theory and game theory to arrive at predictions about what elite decision-making groups are likely to do in various scenarios.
He might be right. He is a brilliant and prolific political scientist. But we are from Missouri. We want to discover the answers to three categories of questions:
- What happens when we pit Bueno de Mesquita’s approach against formidable competition on a level playing field under the control of a neutral respected arbiter (such as IARPA?) that is committed to making all data public and to laying out the scoring rules in advance;
- Can we decompose his approach into its components? Decomposition of his multistage prediction generation machine is crucial for identifying where the greatest value is added. For instance, it is one thing to say that his method outperforms 80% of the individual area-study experts who provide the informational inputs to the algorithms. It is quite another to show that the machine can outperform the average forecast of the same experts, the infamous wisdom of the crowd effect. After all, we know that averaged forecasts are often better than 80% or more of the individuals from whom the averages were derived.
- Can we ensure that Bueno de Mesquita’s system is indeed pitted against the most formidable competitors in academia and the private sector? As already noted above, simple averaging of experts is often a very tough benchmark to beat. And it is easy to imagine refinements that might be tougher—such as giving greater weight to forecasters with a track record of being better calibrated or greater weight to forecasters who have been given various forms of training in probabilistic reasoning or forecasters who are interacting in prediction markets or other types of social systems for information aggregation.
Again, we do not know which hypotheses will fare well and which will bite the dust. But we are glad to share our current best guess. For many categories of forecasting problems, we are likely to bump into the optimal forecasting frontier quite quickly. There is an irreducible indeterminacy to history and no amount of ingenuity will allow us to predict beyond a certain point. But no one knows exactly where that point lies. And it is worth finding out in as dispassionate and non-ideological fashion as we human beings are capable of mustering.
IARPA and Open Testing Methods
I find myself overwhelmingly in agreement with Gardner and Tetlock. The IARPA undertaking may well be the right and most opportune forum for testing alternative theories, methods, and hunches about prediction. Just tell me how to participate and I am happy to do so. I have already provided Tetlock with dozens of forecasts by my students over the past two or three years. These are ready for comparison to other predictions/analyses of the same events. What I don’t know is what questions IARPA wants forecasts on and whether those questions are structured in a way suitable to my method. My method requires an issue continuum (it can handle dichotomous choices but those tend to be uninteresting as they leave no space for compromise), specification of players interested in influencing the outcome, their current bargaining positions, salience, flexibility and potential clout. Preferences are assumed to be single-peaked and players are assumed to update/learn in accordance with Bayes’ Rule. Bring on the questions and let’s start testing.
As for decomposing my model, the information to do so is pretty much all in print so no problem there although the nature of game theoretic reasoning is that the parts are interactive, not independent and additive so I am not sure exactly what is to be advanced by decomposition. But I am happy to leave that to impartial arbiters. Perhaps what Gardner and Tetlock have in mind is testing not only the predicted policy outcome on issues, but also the model’s predictions about the trajectory that gets it to the equilibrium result: how do player positions, influence, salience and flexibility change over time for instance. As long as they have the resources to do the evaluation, great!